An Argument for Modeling Consolidation
Or What Happens if Attention (and Transformer) Is All We Need?
✋ This post uses images, so if you are reading this on an email client, enable the “display images from sender” to get the most out of the post. If you are a new subscriber, welcome! If you find these writings valuable, consider sharing them with a colleague or giving a shoutout on Twitter.
What if a Transformer is all we need?
Even if you don’t believe in that question, let’s consider it for a moment.
There are reasons for even such a consideration. In less than five years, no AI application area has been untouched by the transformer, in particular, and attention-based models in general. The fire that started in NLP has moved beyond and burned into computer vision, speech, computational biology, reinforcement learning, and robotics, decimating all competition in its path.
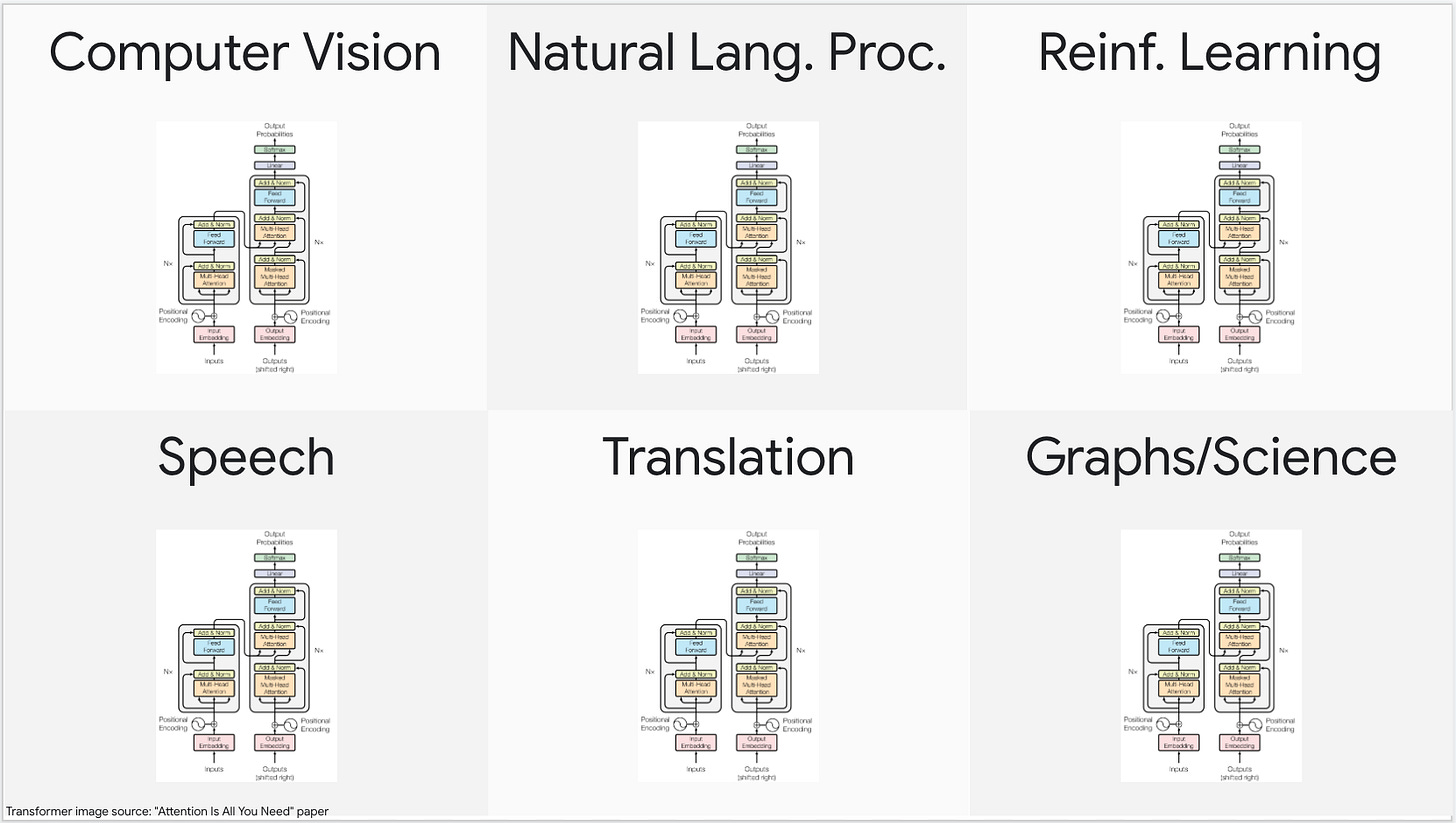
Andrej Karpathy made an astute observation about the consolidation of ML methods and architectures that goes something like this (my paraphrase, for brevity):
Since 2012, Autodiff-driven backpropagation has more or less replaced a zoo of machine learning methods. This led to a zoo of DL architectures, and since 2017 most of these alternative architectures have been subsumed by one architecture — The Transformer.
In my ~20 years of experience with AI, I haven’t experienced this kind of consolidation and performance. I can almost hear some of the readers revolting against my use of “performance” here. Yes, the transformer models still have some ways to go. But the good news is that we have turned, in many cases, problems that were impossible into engineering problems where we know how to measure, optimize, and iterate. So I expect every aspect of the transformer modeling pipeline to get optimized within the next 2-3 years.
The “hardware lottery” camp might view this as an adverse event, but here’s the deal: Most researchers are not builders. Researchers are incentivized to explore and, thus, have an exploration bias. Builders, on the other hand, have an exploitation bias. For further criticism of the hardware lottery paper, see my post on riding the hardware lottery.
That said, the search for a grand unified architecture that can model all data is still on (Graph Neural Networks or GNNs have made some strides here), and I am not claiming transformers are it. But instead, I am considering the hypothetical of “what if” transformers, particularly very large versions of them, happen to provide this unification. How will that future unfold? If you are a builder, where should you focus the most? If you are an investor, how will you write your thesis for AI companies software any company?
1) We will see a dramatic spread of research ideas from not just one area of AI to another but from one area of science to another. Say a grad student in an astronomy lab comes up with a new way of doing position embedding in transformers for large datasets and puts that on arXiv. The next day, a biologist can read and understand that paper (because we are now speaking the same language) and implement it in her work. This kind of unification will not just catalyze the spread of ideas, but we will see catalysis of progress in all AI-driven fields, which currently is all of STEM and arts. We will witness progress in humanity that was only preceded by meta-inventions like steam engines and electricity.
2) Consistent high performance across multiple modalities and unified representations will make the transformer the model of choice for combining data from different modalities, leading to a proliferation of multimodal applications, putting AR and VR in the front center.
3) Consistent OODA optimizations on different parts of the transformer pipeline mean standard and exchangeable interfaces and more competition between providers, leading to ever-decreasing costs for end users.
4) An exciting category of cost is the ease of use. This consolidation will enable simpler interfaces that can bring diverse people with ideas to participate in building software applications for everyone. Companies building infrastructure that enables this very early on will see winner-take-all effects.
5) A surprising effect of this consolidation and transformer performance becoming “too good to ignore” is that companies and research teams will be forced to work on explaining model predictions. Hence, there will be a massive uptick in model explainability work. The ground zero companies for model explainability are yet to be built. Explainability is one area I am excited about and will undoubtedly be the subject of future issues.
6) As the cost and the direct involvement of builders with modeling go down, we can anticipate more investments in pre- and post-modeling parts of the pipeline, which are typically domain-specific. Perhaps consolidation might be what we need to get engineers out of their modeling sandboxes and back to product and customer needs.
7) Security, bias, and other issues around transformer models will be inevitable as their proliferation continues (this is true for all modeling). However, consolidation means the pressure to fix problems as they arise will also increase, resulting in increased investment in the number of researchers and engineers working on those problems.
Of course, the future never unfolds linearly, as presented here. Considering the history of technology and engineering, consolidation of ideas is a given. I am not saying Transformers will be the future of modeling (some claim it already), but if there ever was a candidate for that, transformers certainly would be one. Also, consolidation doesn’t mean other ideas will die out; just that resources for ideas will be distributed according to a power law. But it’s interesting to start thinking about the second and third-order effects of modeling consolidation and prepare accordingly.
Like your observation on the consolidation of architectures due to the transformer. It would be cool if we had a new wave of startups focussing on optimizations (software & hardware) to bring down the near-quadratic complexity of this architecture.